TrueHoop projections: Players set to explode
And…surprise! Your favorite player will probably play a little worse this season.
There has never been a better time to subscribe to TrueHoop!
BY HENRY ABBOTT
During last year’s playoffs, David Thorpe used a word that succinctly captured something for me. The Raptors had some players who, as he put it, “accelerated.” As in, Fred VanVleet and Pascal Siakam had careers on a certain trajectory and then kicked them into another gear. Accelerators are easy to find on title rosters and, I’d argue, a good reason to A) have multiple high-potential young players around and B) seek a coaching staff with a better-than-average history of finding the gas pedal.
So I asked David to use his keen eye to predict the NBA players who are most likely to accelerate this season.
Thorpe’s top picks to improve in 2019-2020:
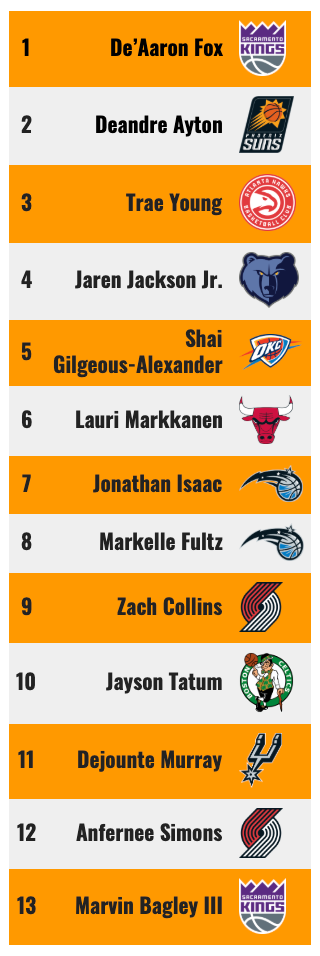
Honorable mentions:
Jaylen Brown, Bam Adebayo, Mitchell Robinson, Ben Simmons, Pascal Siakam, Collin Sexton, Domantas Sabonis, Myles Turner, John Collins, Dwayne Bacon, Justise Winslow, Lonzo Ball, Brandon Ingram, Lonnie Walker IV, Kevon Looney, Willie Cauley-Stein, Ivica Zubac, Harry Giles III
David is a basketball genius, and I couldn’t have more confidence in his picks, but it seemed responsible to contextualize them with some data. If we did a sober analysis of typical historical trends, who would you expect to improve most? Twenty-one-year-old guard Trae Young, who can score like the wind but hasn’t been able to play effective defense? Or 23-year-old Ben Simmons who can basically do everything except shoot?
People have put together papers on such data. Five years ago, Harrison Chase—then at Harvard—was one of those people. Now he’s a machine-learning expert at a Boston-area company called Kensho. Chase did a ton of work with fellow machine-learning expert Anthony Liu to help us make educated guesses on our “Most Likely to Accelerate” list (scroll down to the end of this story for a discussion of their sophisticated methodology).
In a nutshell, Chase and Liu built smart models to predict a player’s Box Plus-Minus (BPM)—similar to Real Plus-Minus—in the upcoming season. The main factors they considered were last year’s BPM, age, and minutes played. “We also included a bunch of other advanced stats (Win Shares, true shooting percentage, effective field goal percentage, etc.),” they explained by email, “which we hoped would stabilize some of the variability of BPM. Interestingly, playoff performance by itself also shows up as a helpful variable, suggesting strong postseason performance could be a sign of good things to come in the next season.”
I first went to Chase and Liu wondering which players would improve the most, thinking their list might end up similar to David’s. But what I got was a hard lesson in regression to the mean. In short: ALL of the players predicted to have huge improvements were so bad last year that even after amazing acceleration, projections for this year still have them hurting their teams every second they are on the floor. In the days to come the whole list will be public, and you’ll be able to explore for yourself. But for now, take my word for it that not one of the projected huge improvers even meets the BPM level of -2, which is a replacement-level player. So the list feels kind of…tangential to this season.
So we looked at it another way: who will improve the most out of those projected to be pretty good? In the end, I think that’s how Thorpe and I always interpreted the question.
Arbitrarily, I decided to consider “good” players to be at -1 or better, a little better than replacement level. Among those players, I only wanted players slated to improve.
Here’s the big surprising news: There are only 13 players in the whole league who fit that description. Incredible, huh?
Projected to improve most in BPM in 2019-2020, among “good” players:
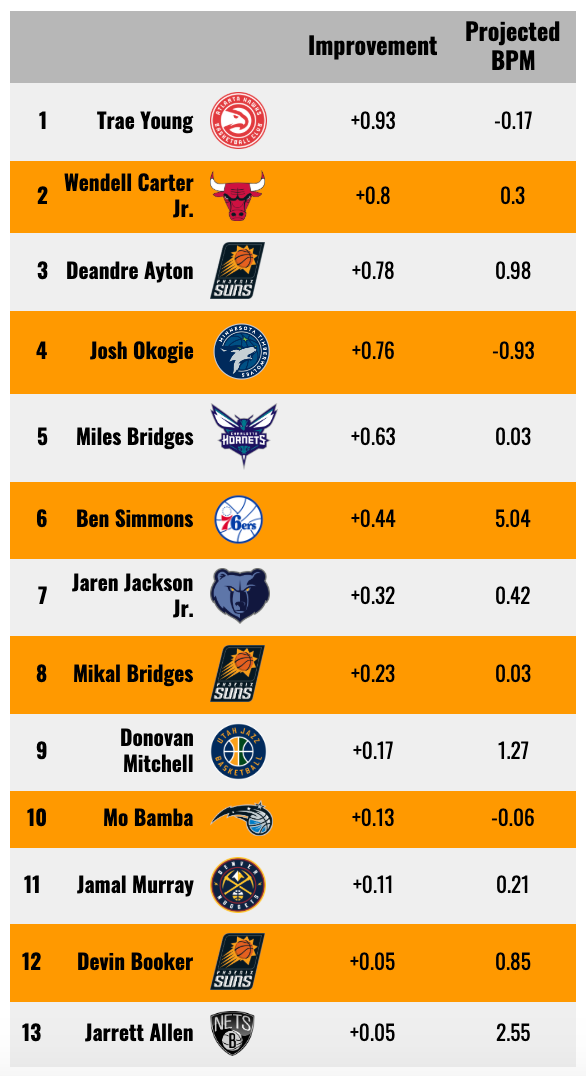
Once you get over the reality that the numbers say most of your dreams for this season won’t come true—most players are not due to explode—there’s a lot to like here.
For one thing, the NBA has close to 400 players. Thorpe made his list weeks ago. The machine learning happened with no knowledge of Thorpe’s picks. Yet somehow both lists include Trae Young, Deandre Ayton, Ben Simmons, and Jaren Jackson, Jr. Cool!
I also like that a lot of the names are surprising. (Every player named “Bridges!”) Chase and Liu point out that BPM has a known tendency to highly value (maybe even over-value) big men who log lots of blocks and rebounds. So there are a lot of Mo Bambas. Jarrett Allen is projected to be far more valuable than, say, Jamal Murray.
The big news here, though, is that by this metric, Ben Simmons is the NBA’s only seriously productive player who is slated to improve at all this year.
Let that sink in.
ESPN just published #NBArank. The top player in basketball is said to be Giannis Antetokounmpo. Chase and Liu’s model projects he’ll be a tad worse than last year. Second-ranked by ESPN is Kawhi Leonard, who is also slated for a bit of backsliding. That’s true of every single player in the top ten—as well as everyone in the top 20 who is not named Ben Simmons or Donovan Mitchell. Sorry, Nikola Jokic, Joel Embiid, and Luka Doncic. These are ESPN’s top 20, with arrows showing their predicted productivity this season:
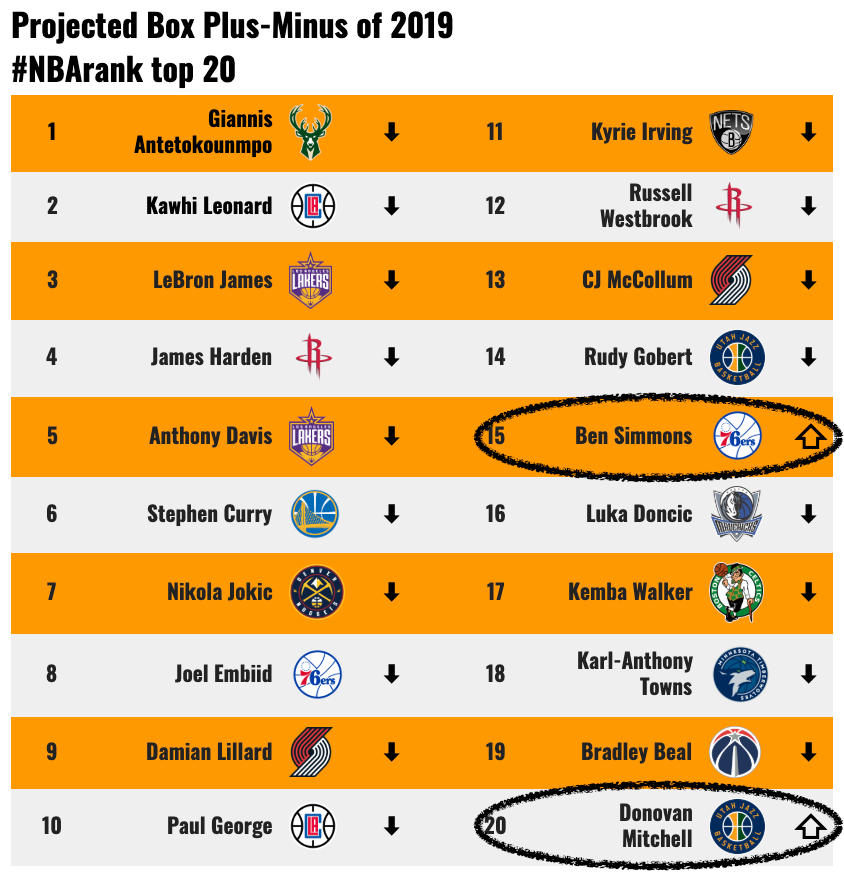
“Honestly, not terribly surprising,” email Chase and Liu. “Our model is fairly conservative, and one of the main things the model learned was some type of regression to the mean. That means that on average, players who are toward the top of the league in one season are there because they outperformed their skill level. After that, we often see regression of some sort the following season. Consider this fact: if you take the top 20 players in BPM for each of the past five seasons (with above 1000 minutes, so we get rid of players with a small sample size), only 33 percent had a higher BPM the following season! This isn’t just a recent thing either. If you go back fifteen years, that number drops to 30 percent. We’re not the only ones to notice this trend. If you look at CARMELO, FiveThirtyEight’s projection system, top players like Harden, Curry, Jokic, and Giannis all have projected drops from their previous years.”
A lot of it has to do with age. Few of the NBA’s most famous and popular players are particularly young. Becoming famous takes a few years! And those are the years when players improve the most. “Serious improvements past the age of 25 are rare to find,” Chase and Liu said. That might, in fact, be the key finding of the project. Age counts. It shouldn’t be surprising since the data has always screamed that very thing. “If you look at the 100 players in our dataset who improved the most over their previous seasons while posting a positive BPM, 67 of them were 24 or younger. Although older players can definitely make the leap if put in the right scenario, we don’t have a ton of complex features to capture these ‘right’ scenarios—and so it is not terribly surprising that, on average, our model projects younger players improve the most.”
HOW THESE PROJECTIONS WERE MADE
Talking to Kensho’s Harrison Chase and Tony Liu
Can you give a plain English description of the process?
We set an end goal of trying to predict a player’s BPM for the upcoming year. Let’s first talk about the target, and then the approach. We chose BPM as the target. BPM (Box-Plus Minus) is an all-in-one stat readily available on Basketball Reference, where there is a thorough explanation. Although there are known issues with this stat (see later discussion points), we chose it for several reasons. Primarily, it’s easy to get historical values for BPM, and a bonus of being on Basketball Reference that it’s linked to plenty of other data. We decided to build one model (Model A), which tried to predict BPM directly. We also built a separate model (Model B), which instead tried to predict a player’s change in BPM from the previous year. Then we blended the results of both models to arrive at our final predictions (unless the player had not played the year before, in which case we used only Model A). For all models, we used LightGBM, a gradient boosting framework, which is good because it has state-of-the-art-results on most prediction problems. The downside is that it’s a bit tougher to explain some of our predictions.
What are the inputs? Why do we believe in them?
The inputs that seemed to carry the most weight were the players’ historical BPM and age. These make a ton of sense: How a player does the year before should be a pretty good indicator of how they do the next year. And, intuitively, age is probably the most consistent predictor of whether players will improve or decline (e.g., older players are more likely to get worse).
Besides these inputs, we found minutes played to be an important variable. This makes sense for several reasons: First, the more minutes played last year, the more stable our measurement of their ability (through BPM). Second, more minutes played could mean the coach viewed this player as better than their BPM reflected (or as having potential), and their BPM could make a jump to expected levels next year. Finally, for young players, more minutes played just means more chances to improve and get better.
We also included many other advanced stats (win shares, true shooting percentage, effective field goal percentage, etc.), hoping to stabilize some of the variability of BPM. Interestingly, playoff performance by itself also shows up as a helpful variable, suggesting strong performance in the postseason could be a sign of good things to come in the next season. We found other external factors like team play important, like whether the player stays on the same team, and how many minutes teammates of his position played last year.
We saw that players who changed teams were more likely to see a drop in BPM. We also saw that when a player’s teammates at the same position did not play a lot of minutes last year, he was more likely to see an increase in BPM. We hypothesize this means that when a starting shooting guard leaves a team, and no replacement is brought in, others at that position will have a chance to shine. Conversely, adding an elite shooting guard to a team means others will have their minutes and workload reduced.
What did you learn from your earlier work—for instance with production curves—that informs this?
One big thing we learned from earlier work is that modeling changes in BPM (rather than BPM directly) can be better in certain cases. However, we had also seen instances when it was definitely NOT always better, and in extreme cases, could lead to wacky values. Blending the two predictions yielded better out of sample predictions. We also included some features around postseason performance from the year prior, as previously we had found that to help (slightly) in predicting player development.
What are the units? How should people looking at this feel about +1 or -5? What does that mean on the court?
For a more thorough definition see Basketball-Reference, but basically, it is a measure of a player’s performance relative to league average on a per 100 possessions scale. +5 is roughly all NBA level, -2 is replacement level, -5 is really bad.
What did you expect to find, and how did that compare with what you actually found?
The largest variables (BPM the year before and age) were pretty expected, nothing too shocking there. We also expected to find a lot of regression to mean—and we found it! This did not really surprise us—the best players any given year are usually the best because they’ve had really good seasons, and so will likely revert a bit next year. This is especially true if you are looking at a stat like BPM (or any all-in-one stat, really), which seems to be change year-to-year quite a bit.
What was the biggest surprise?
There were a bunch of surprises. For variables that we thought would help but didn’t: MVP/MIP/ROY awardshare from the year before, coaching stability, and usage rate. Variables showing a stronger effect than expected are playoff performance, and the minutes played by teammates of same position during the previous year.
How do you feel about the outcome?
Pretty good, especially given the time constraints! I think we managed to include most of the obvious features, and had time to experiment with a few more elaborate ones. Some downsides of the model are that the factors we included are a lot simpler than the insights an analyst might come up with. This is because analyst insights are usually more complex and intricate, building predictions off of input variables that take time to put together and are just really hard to include in a model. For example, a recent Ringer piece highlights Josh Richardson as one player who made a leap based on compatibility with his new team. Player compatibility in the NBA is a tricky thing to measure, probably worth its own deep dive. Creating something like that as an input for this model was not feasible given the time constraints. However, it would no doubt be fun to try to incorporate the approaches of other writers in predicting which players they expected to take a leap, and to try to include the evidence they point to as features in our own work. Perhaps next year!
A general observation: The projected huge improvements are almost all, essentially, terrible players projected to hurt their teams less. Fair?
Yes! This comes back to regression to the mean and small sample size. A lot of the worst players by BPM did not play many minutes, and BPM can change a lot year to year for players that don’t get a ton of reps. Also, if a player with a terrible BPM is brought back to the league, that usually means the team expects him to get better (either because he is young or because he is unlucky), so this leads to a bit of bias in the data. Bad players either 1) don’t come back, so we don’t have any data for them, or 2) come back (usually because they performed worse than expected the year before) and seem to improve the next year.
It looks like almost every All-Star or huge contract player is slated to be a little worse next year than this year. This feels like a fascinating finding. How do you take that?
Honestly, not terribly surprising. Our model is fairly conservative, and one of the main things the model learned was some type of regression to the mean. On average, players who are toward the top of the league in one season are there because they really outperformed their skill level, and we often see regression of some sort the next season. Consider this fact: if you take the top 20 players in BPM for each of the past five seasons (with above 1000 minutes, so we get rid of players with a small sample size), only 33 percent had a higher BPM the following season! This isn’t just a recent thing either--if you go back fifteen years, that number drops to 30 percent. And we’re not the only ones to notice this trend. If you look at CARMELO, FiveThirtyEight’s projection system, top players like Harden, Curry, Jokic, and Giannis all have projected drops from their previous years.
Is there any truly effective NBA player for whom this model projects a big improvement?
A few! Ben Simmons and Donovan Mitchell are projected to have career years. Andre Drummond and Draymond Green are expected to have bounce-back years (albeit not career years). Overall though, our model is definitely on the conservative side of things; it will be rare for it to predict any large jumps for players that already have high BPM.
I suppose we should talk about Joe Chealey.
Hah! I suppose you are referring to the fact that he is expected to jump up 12 (!!) points in BPM. I think it’s important to keep in mind several things. First, his BPM last year was abysmal: -17. Second, he only played 8 minutes last year, so if there were error bars for BPM estimates, his would be huge. Lastly, he is still projected to be -4.8, so still a pretty bad player.
I find myself interested to know "of players projected to actually help their teams next year, who do we expect to improve the most?" That list is fascinating, and thick with names like Wendell Carter Jr., Deandre Ayton, Ben Simmons, Donovan Mitchell, Jamal Murray, Devin Booker, Zach Collins, Jonathan Isaac. Those people I just named were all born between 1996 and 1999!
Not terribly surprising! If you look at the data, overwhelmingly younger players improve the most. Serious improvements past the age of 25 are rare to find. If you look at the 100 players in our dataset who improved the most over their previous seasons while posting a positive BPM, 67 of them were 24 or younger. Although older players can definitely make the leap if put in the right scenario, we don’t have a ton of complex features to capture these “right” scenarios—and so it stands to reason that on average, our model projects players in that age span to improve the most.
You pointed out that there is something going on with big men. Can you explain that a bit, and maybe help us think about how to apply that knowledge to these results?
So I think the key thing to keep in mind—not only here but for this whole exercise—is that this relies extremely heavily on BPM. And although BPM is great for a lot of reasons, it can also be bad for a lot of reasons. As touched on before, it can be unstable. It can also overvalue or undervalue certain things, as opposed to both of the other all-in-one metrics and our own eye test. One of those things seems to be rebounds/blocks, as it seemed to us that players like Rudy Gobert, Nikola Vucevic, Jusuf Nurkic, Derrick Favors, etc. may be overrated. This means that when predictions appear to overvalue or undervalue a player, there is a good chance that means that BPM overvalues or undervalues said player, not our model.
Thank you for subscribing to TrueHoop! Next week: we dig deeper into the data, and how it affects your team.